
Untangling tangles: a new approach to the problem of community identification
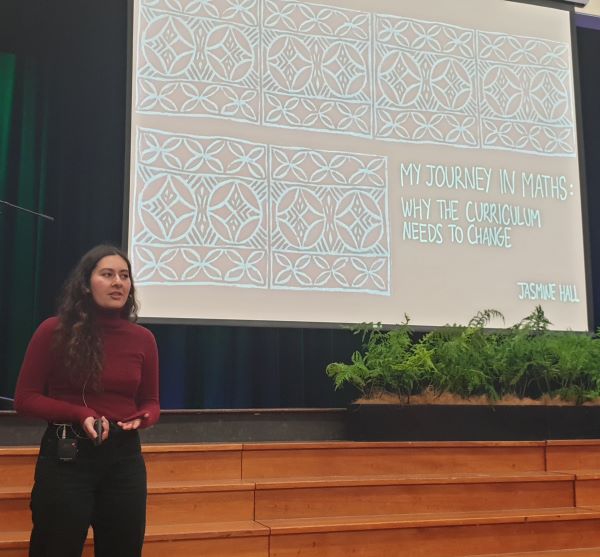
Dr Jasmine Hall (photo supplied).
We live in a world run by big data. From the targeted social-media advertising employed in Trump’s election campaigns, to fraud detection, to self-driving cars – many of our daily interactions rely on big data, and the implications are huge. Big data, in its nature, is unwieldy, but once it is processed, analysed and understood, its value and potential are limitless. The community identification problem is a fundamental problem in big data. Consider Trump’s targeted campaigning relied on identifying communities sympathetic to his policies. How do we determine whether a person ‘belongs’ to a certain community or not? Communities are not generally easily defined, which makes the problem of community identification so difficult. The need for a mathematically robust approach is immense. Our innovative approach is to utilize structures called tangles. Given any data set, finding the tangles means finding the communities. Not only are tangles a genuinely robust method for identifying communities, but a distinguishing feature is that they embrace the inherent fuzziness which exists on community boundaries. However, there is a cost associated with tangles. As the research currently stands, we can’t identify the tangles (and therefore the communities) of a data set easily or quickly: the problem of tangle identification is exponential in complexity. This project builds on collaborative work with Semple and Whittle, in which we brought tractability to the tangle identification problem by defining a special class of tangles, which in contrast to general tangles, can be found both quickly and easily in polynomial time. The goals of this project are twofold: firstly, to deepen our understanding of this special class of tangles and secondly, to determine the feasibility of our techniques to the problem of community identification. I will also continue my research in mathematics communication and in the Pacific space.